Getting Reality Right

Excerpt from the book: Systems Thinking Made Simple, Chapter 2
The British mathematician George E.P. Box said, "All mental models are wrong; the practical question is how wrong do they have to be to not be useful." It's a simple statement with profound implications. It means that everything we think about the systems around us is merely an approximation. Our mental models are always wrong in the sense that they never completely capture the complexities of the real world. But mental models are useful because sometimes they get it "right enough." The Nobel laureate Herbert Simon coined the term satisfying and contrasted it with optimizing. Simon explained that organisms of all kinds may avoid the costs associated with optimization (since perfection is expensive) and instead try to find a satisfactory solution. They're okay with good enough because it gets the job done.
In his book, Thinking, Fast and Slow, Nobel laureate and psychologist Daniel Kahneman describes his research on two modes of thought he calls System 1 and System 2. He writes, "System 1 operates automatically and quickly, with little or no effort and no sense of voluntary control. System 2 allocates attention to the effortful mental activities that demand it, including complex computations." His purpose is to show how biased our human judgment can be. System 1 is evolutionarily valuable because although it's often wrong, it's right enough (i.e., cognitive satisfying) and has the added advantage of being fast. System 2 is more accurate but takes longer. System 1 works reasonably well when the problems humans deal with are routine, familiar, tangible, rudimentary, and simple (i.e., the kind of problems we might experience in hunter/gatherer, agrarian, or industrial ages). But as society becomes more complex and abstract, with more information, interconnections, perspectives, and systemic effects, the relative accuracy of System 1's mental models declines. Also, the amount of time we have to think about complex problems shrinks. What we need is System 2 accuracy with the speed of System 1. As the stakes get higher, it becomes even more important that our mental models reflect reality. The four simple rules of systems thinking that you will learn more about in chapter 3 provide a basis for better understanding System 1 and System 2 processes and increasing their accuracy and speed, respectively.
Figure 2.7 shows the relationships among the variables we are dealing with as we attempt to solve real-world wicked problems. We create mental models that summarize and are capable of describing, predicting, and altering behavior. In other words, our mental models may lead us to think certain things about the real world and result in actual behaviors in the real world. These predictions, descriptions, or behaviors lead to real-world consequences that in turn provide feedback or data that inform our mental models. If we are paying attention, this feedback helps us adapt and select the best mental models. Ideally, we want our mental models to reflect the salient aspects of the real-world system or problem we are trying to solve. The way we know whether or not our mental model is right (or at least satisfies) is that we try it out in the real world and see what happens. If what we expect to occur occurs, then the feedback we receive from the real world tells us our mental model is well constructed. If we expect something to occur and it doesn't, then the feedback we receive from the real world tells us our model needs some work. Either way, we (hopefully) take in this new data which informs what is (hopefully) a continuous process of mental model improvement.
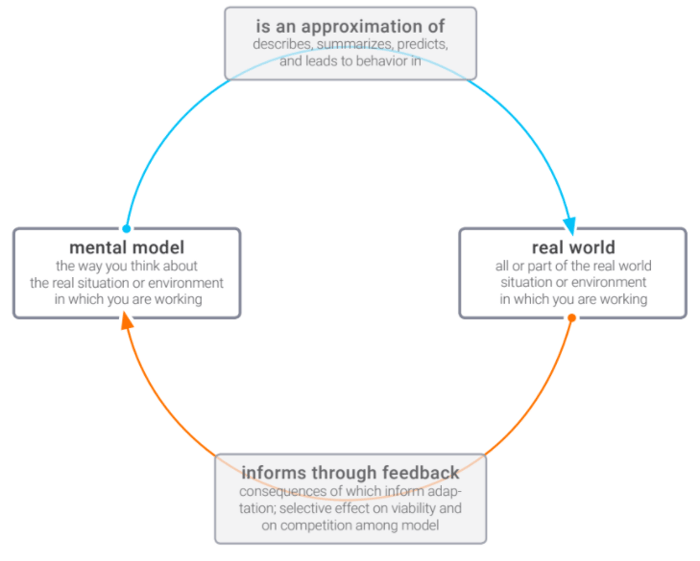
But the basic process described in Figure 2.7 need not be thought of as purely a mental, cognitive, or conceptual process. It describes a process that has many synonyms that at first we might not perceive as such. Figure 2.7 also describes the processes of learning, evolution, feedback, adaptation, knowledge, science, and complex adaptive systems.
For example:
- Individual learning: a process of building mental models and adapting them to the environment;
- Organizational learning: a process of sharing mental models across individuals and adapting the mental models to the environment;
- Feedback: the process of acting upon and reacting to a stimulus, environment, or system;
- Adaptation: the process of changing to become better suited to one's environment;
- Complex adaptive system (CAS): a system that adapts to become better suited to its environment;
- Science: a process of building mental models (knowledge) that better approximate reality; and
- Knowledge: the collection of individual or shared mental models used to navigate the real world.
If we simply exchange the term mental model with schema (a more general term that includes physical, chemical, biological, mental, or any other types of models) you can see that the model need not be a conceptual one, but could instead be a genetic model such as the DNA sequence of a platypus. Like mental models which represent a hypothesis, every organism and species is also hypothesis—a genetic model—that may or may not turn out to be viable, and therefore experience selective effects and compete with other models (a.k.a., evolution). A mutation is in one sense a model or hypothesis that may turn out to be adaptive or maladaptive. If ants live in deep tiny holes and adaptations lead to longer, pointier beaks in birds, then the model turns out to be adaptive, whereas the competing pancake-shaped beak model may turn out to be maladaptive.
Figure 2.7 describes learning as a global phenomenon as well as the basic structure of all evolutionary processes where things adapt to their environment. Figure 2.7 also describes learning at the societal level where science is an adaptive process of building mental models (knowledge) that better approximate reality.
These phenomena that may at first seem very different are structurally similar underneath. Each of these phenomena fundamentally involves a model or schema that gets tested against the real world. Selection pressures affect the viability of any model against competing models. There are winners and losers. Science works the same way. We come up with hypotheses, concepts, models, or theories and test them against the real world and see which ones win and lose. Learning, both individual and organizational, entails the development of mental models; the ones that work (for some purpose which is often not conscious to us at the time) survive. Those that don't work perish. Of course, this can be quite complex as you might develop mental models with the purpose of keeping yourself in denial, because the truth is simply too difficult to handle. You test the model in reality, and if it works, the model is preserved. So the purpose of the model is often important and the purpose is not always about getting closer to the truth.
Figure 2.7 depicts how complex adaptive systems work, and describes the learning process that makes a complex adaptive system adaptive. The process that allows it to adapt to its environment is that it learns. Figure 2.7 just as easily characterizes all forms of adaptive knowing and learning. That is, it is the model of science itself. Remember, systems thinking is all about increasing the probability of getting the mental model right! Here "right" means that it better approximates the real world.
Individual survival is based on this feedback loop between mental models and the real world. But whole organizations and civilizations rely on it, too. Every organization's survival depends on its ability to learn. CEO Jack Welch said it this way: "An organization's ability to learn, and translate that learning into action rapidly, is the ultimate competitive advantage." So this model is critical. We want you to understand it so well that its basic structure is burned into your mind because it is the basis of systems thinking: that the mismatch between how real-world systems work and how we think they work leads to wicked problems. We want you to take another look at Figure 2.7, understand it, and then shrink it down into a little representational picture like this:
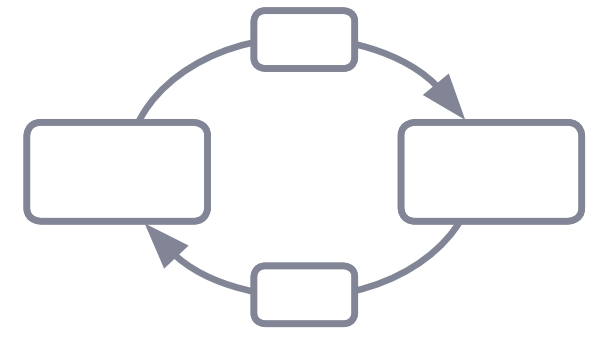
We can even shrink it down further into what we call a sparkmap , which is a tiny, structural mental model that sparks prior understanding and can be situated in text.
Murray Gell-Mann is one of the greatest minds you've probably never heard of. His scientific achievements include decades of innovation; he gave us insight into the quantum world, for which he won a Nobel Prize. Gell-Mann, along with other great minds, founded the Santa Fe Institute for the study of complex systems (SFI), which has significantly advanced the field of complexity science. Figure 2.9 is a diagram he developed that gives a big-picture view of what he calls "Some complex adaptive systems on Earth."
We'll start at the bottom, in the primordial soup, where pre-lifelike chemical reactions are subject to evolutionary pressures. Out of these processes emerges the biological evolution of both organisms and ecosystems. From this in turn emerges both the mammalian immune system and individual learning and thinking. This is an important step because this is where systems thinking lives. So pay particular attention to the red parts of the diagram [emphasis ours]. It is through the transmission of learning among individuals (both within and across generations) that human culture emerges. This is critical, because individual thinking and learning is at the root of the creation of culture (i.e., sharing mental models with others).
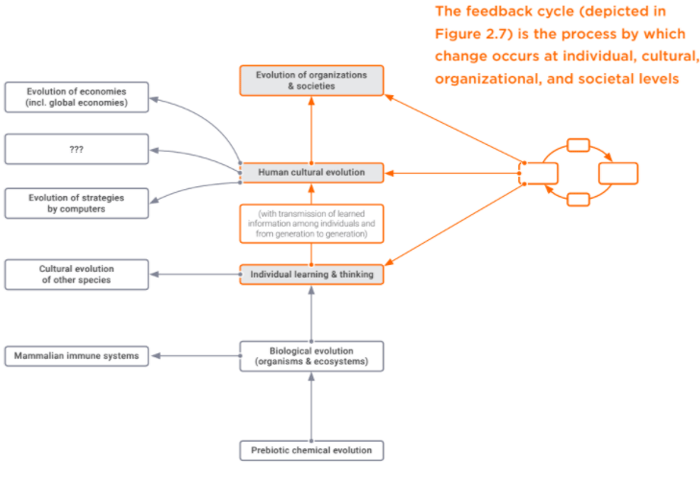
At the cultural and organizational levels, the same learning feedback loop occurs but for the larger group or organization. Of course, this same culture-building process leads to the emergence of economies, technologies, and other phenomena on a mass scale.